DeepLabCut
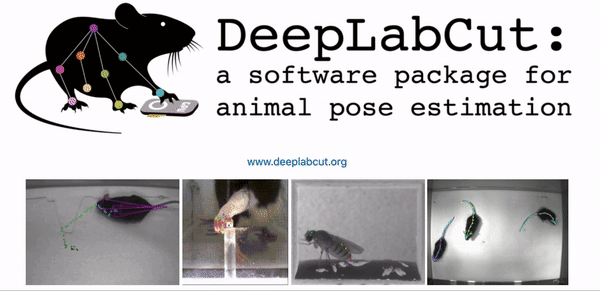
DeepLabCut™ is an efficient method for 3D markerless pose estimation based on transfer learning with deep neural networks that achieves excellent results (i.e. you can match human labeling accuracy) with minimal training data (typically 50-200 frames). We demonstrate the versatility of this framework by tracking various body parts in multiple species across a broad collection of behaviors.
The package is open source, fast, robust, and can be used to compute 3D pose estimates. Please see the original paper and the latest work below. This package is collaboratively developed by the Mathis Group & Mathis Lab at EPFL/Harvard.
The code is freely available and easy to install in a few clicks with Anaconda (and pypi). Please see instructions on deeplabcut.org. We also provide a very easy to use GUI interface, and a step-by-step user guide!
Project Author(s)
Mackenzie Mathis, Alexander Mathis & contributors
Project Links
Project Video
https://www.youtube.com/channel/UC2HEbWpC_1v6i9RnDMy-dfA
This post was automatically generated by Mackenzie Mathis