YAPiC
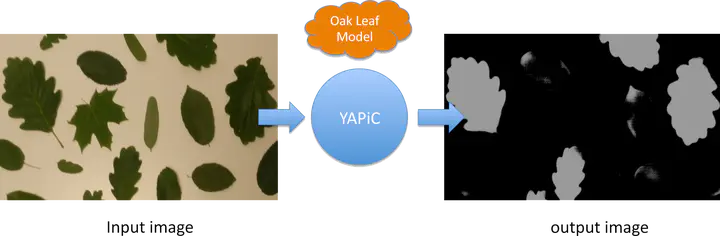
World Wide Series Seminar
With YAPiC you can make your own customized filter (also called model or classifier) to enhance a certain structure of your choice with a simple Python based command line interface, installable with pip. We have used YAPiC so far for analyzing various microscopy image data. Our experiments are mainly related to neurobiology, cell biology, histopathology and drug discovery (high content screening). However, YAPiC is a very generally applicable tool and can be applied to very different domains. It could be used for detecting e.g. forest regions in satellite images, clouds in landscape photographs or fried eggs in food photography. Pixel classification in YAPiC is based on deep learning wit fully convolutional neural networks. Development of YAPiC started in 2015, when Ronneberger et al. presented a U-shaped fully convolutional neural network that was capable of solving highly challenging pixel classification tasks in bio images, such as tumor classification in histological slides or cell segmentation in brightfield DIC images.
YAPiC was designed to make this new kind of AI powered pixel classification simply applicable, i.e feasible to use for a PhD student in his/her imaging project.
Project Author(s)
Christoph Moehl; Manuel Schoelling
Project Links
https://yapic.github.io/yapic/
This post was automatically generated by Christoph Moehl